One-Stage-TFS: Thai One-Stage Fingerspelling Dataset
Siriwiwat Lata and Olarik Surinta
The Thai One-Stage Fingerspelling (One-Stage-TFS) dataset is a comprehensive resource designed to advance research in hand gesture recognition, explicitly focusing on the recognition of Thai sign language. This dataset comprises 7,200 images capturing 15 one-stage consonant gestures performed by undergraduate students from Rajabhat Maha Sarakham University, Thailand. The contributors include both expert students from the Special Education Department with proficiency in Thai sign language and students from other departments without prior sign language experience. Images were collected between July and December 2021 using a DSLR camera, with contributors demonstrating hand gestures against both simple and complex backgrounds. The One-Stage-TFS dataset presents challenges in detecting and recognizing hand gestures, offering opportunities to develop novel end-to-end recognition frameworks. Researchers can utilize this dataset to explore deep learning methods for hand detection, followed by feature extraction and recognition using techniques like convolutional neural networks, transformers, and adaptive feature fusion networks. The dataset supports a wide range of applications in computer science, including deep learning, computer vision, and pattern recognition, thereby encouraging further innovation and exploration in these fields.
Keywords:
One-stage fingerspelling; Fingerspelling recognition; Hand detection; Hand gesture recognition; Deep learning; Computer vision
Subject:
Computer Science
Specific subject area:
Fingerspelling recognition is a crucial component of hand gesture recognition frameworks. The Thai One-Stage Fingerspelling Dataset includes images and annotated files that indicate the location of the hand within the images. This dataset is designed to support the detection of hand gestures and the recognition of fingerspelling. Additionally, it is relevant to various fields, including deep learning, computer vision, pattern recognition, and computer science applications.
Type of data:
Image (JPG format) and Raw (XML format)
Data collection:
The Thai One-Stage Fingerspelling (One-Stage-TFS) Dataset provides fingerspelling in the Thai language, focusing exclusively on one-stage consonants. The dataset was collected between July and December 2021 by undergraduate students at Rajabhat Maha Sarakham University, Thailand. The contributors included students from the Special Education Department with experience in Thai sign language and students from other departments without experience.
Data source location:
Institution: Rajabhat Maha Sarakham University
Province: Mahasarakham
Country: Thailand
Related research article:
S. Lata, O. Surinta, An end-to-end Thai fingerspelling recognition framework with deep convolutional neural networks, ICIC Express Letters 16 (2022) 529–536. https://doi.org/10.24507/icicel.16.05.5529
Link to download One-Stage-TFS dataset : https://doi.org/10.17632/rknd3wbz42.1
Cite this dataset: Lata, S., & Surinta, O. (2022). An end-to-end Thai fingerspelling recognition framework with deep convolutional neural networks. ICIC Express Letters, 16(5), 529-536. doi: 10.24507/icicel.16.05.529.

Mulberry Leaf Dataset
Thipwimon Chompookham and Olarik Surinta
The mulberry leaf dataset is a collection of 10 cultivars that are taken in the natural environments using DSLR cameras and smartphones. We collected the data from three regions of Thailand: northern (Chiang Mai), central (Phitsanulok), and northeast (Nakhon Ratchasima, Buriram, and Maha Sarakham).
The mulberry leaf images were captured from the natural environments. We recorded the images from different perspectives. There is a shadow that appears in the photo when holding the camera at a low position. However, when shooting from an eye-level position, the resulting image is sharp and the backlit image does not appear. All leaf images are recorded in the JPEG format.
The mulberry leaf dataset includes ten cultivars, which are four cultivars from Thailand: Chiang Mai 60 (500 images), Buriram 60 (345 images), Kamphaeng Saen 42 (500 images), and 761 images of mixed-breed mulberry (Chiang Mai 60 + Buriram 60). Three cultivars of Australia consist of King Red (350 images), King White (541 images), and Black Australia (637 images). Two cultivars of Taiwan consist of Taiwan Maechor (640 images) and Taiwan Strawberry (488 images). Also, 500 images of the Black Austurkey are from Turkey. This dataset contains 5,262 images in total. Note that mulberry experts advised examination of each mulberry species to label the data and avoid the errors due to the similarity pattern and shape of the leaves.
Link to download Mulberry Leaf dataset : https://drive.google.com/drive/folders/1yuOFMsYapPqWGUq3Wxw6ILn0Koxsm2w9?usp=sharing
Cite this dataset: T. Chompookham and O. Surinta (2021). Ensemble Methods with Deep Convolutional Neural Networks for Plant Leaf Recognition. ICIC Express Letters, 15(6), 553-565.
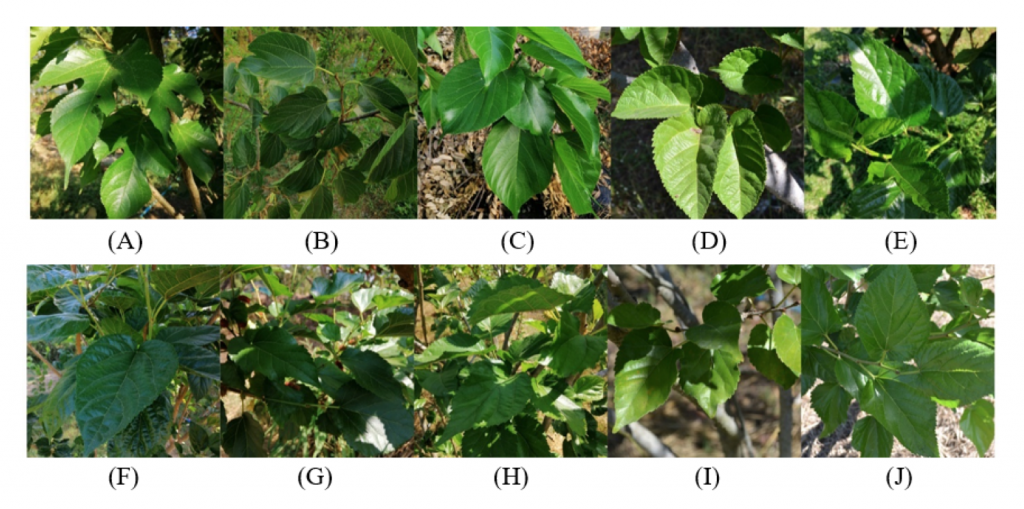
AIWR Dataset
Aerial Image Water Resources Dataset
Sangdaow Noppitak and Olarik Surinta
According to the standard of land use code by fundamental geographic data set: FGDS, Thailand land use classification requires an analysis and transformation of satellite images data together with field survey data. In this article, researchers studied only land use in water bodies. The water bodies in this research can be divided into 2 levels: natural body of water (W1) artificial body of (W2) water.
The aerial image data used in this research was 1:50 meters. Every aerial image had 650×650 pixels. Those images included water bodies type W1 and W2. Ground truth of all aerial images was set for before sending it to be analyzed and interpreted by remote sensing experts. This assured that the water bodies groupings were correct. An example of ground truth, which has been checked by experts. Ground truth has been used in learning the algorithm in deep learning mode and also used in further evaluation.
The aerial images used in the experiment consists of water body: types W1 and W2. Aerial image water resources dataset, AIWR has 800 images. Data were chosen at random and divided into 3 sections: training, validation, and test set with ratio 8:1:1. Therefore, 640 aerial images were used for learning and creating the model, 80 images were used for validation, and the remaining 80 images were used for test.
Link to download AIWR dataset : https://data.mendeley.com/datasets/d73mpc529b/2
Cite this dataset: S. Noppitak, S. Gonwirat, and O. Surinta (2020). Instance Segmentation of Water Body from Aerial Image using Mask Region-based Convolutional Neural Network, in Information Science and System (ICISS), The 3rd International Conference on, 61-66. https://doi.org/10.1145/3388176.3388184
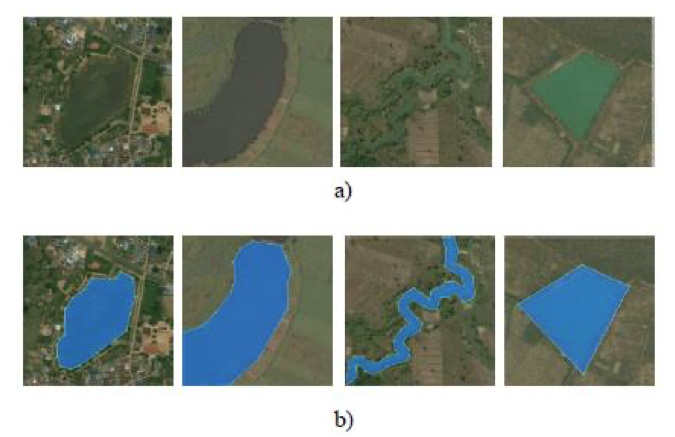
EcoCropsAID Dataset
Thailand’s Economic Crops Aerial Image Dataset
Sangdaow Noppitak and Olarik Surinta
We introduce the novel economic crops aerial image dataset, namely the EcoCropsAID dataset. This dataset was collected in Thailand from five economic crops that were cultivated in different provinces and regions between 2014 and 2018. The aerial images of economic crops were gathered based on Agri-Map Online provided by the Ministry of Agriculture and Cooperatives and the National Electronics and Computer Technology Center (NECTEC). The Agri-Map Online is an agriculture map that all departments under the Ministry of Agriculture and Cooperatives use as an agriculture management tool. Subsequent agricultural information is accurate and up-to-date. Then, the Google Earth application was employed to capture aerial images after we selected the economic crops areas in which images were to be collected. It is quite a complex dataset because the Google Earth program used several remote imaging sensors to record the aerial images.
The EcoCropsAID dataset includes five categories (rice, sugarcane, cassava, rubber, and longan) and contains 5,400 images. Each class has around 1,000 images. To prepare the aerial images of the economic crops, we recorded the image with 600 × 600 pixels and stored it in the RGB color format.
The challenges of classification on the EcoCropsAID dataset are 1) many different image resolutions and colors are contained in the EcoCropsAID dataset due to the various remote imaging sensors, 2) the similarity of patterns amongst each class, for example, longan and rubber, and 3) the difference of pattern inside the same class, for example, cassava and rice.
Link to download EcoCropsAID dataset : https://data.mendeley.com/datasets/g8fhf7fbds
Cite this dataset: S. Noppitak and O. Surinta (2021). Ensemble Convolutional Network Architectures for Land Use Classification in Economic Crops Aerial Images. ICIC Express Letters, 15(6), 531-543.
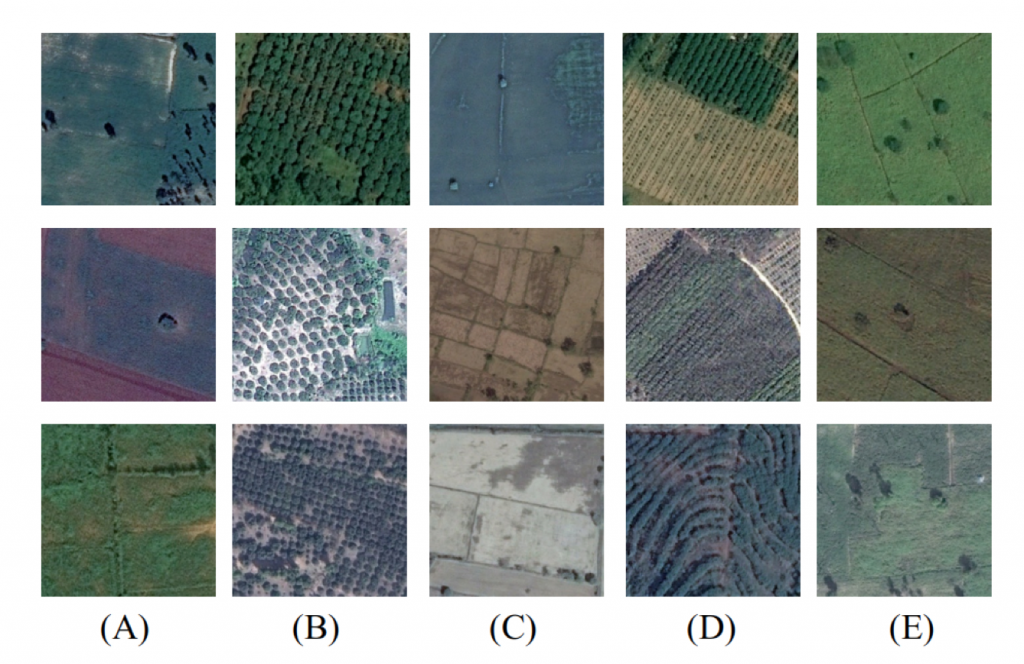
VTID1 Dataset
Vehicle Type Image Dataset (Version 1)
Narong Boonsirisumpun and Olarik Surinta
The main objective for the use of an image dataset was to examine the five vehicle types of motor vehicles that were the most commonly used ones in Thailand (sedan, hatchback, pick-up, SUV, and van). The recording devices to collect the images were part of a video surveillance system located at Loei Rajabhat University in Loei province, Thailand. The collection process took place during the daytime for four weeks between July and December 2018. Two cameras were installed at the front gate of the university. However, a small number of van images was produced in the dataset compared to the number of images of the other four vehicle types. Because of this, the researchers decided to add other vehicle-type images such as those of motorcycles into the van group and changed the name of the group to “other vehicles” to increase diversity. Finally, the first dataset called “Vehicle Type Image Dataset (VTID)” had a total of 1,410 images that could be separated into vehicle types as follows; 400 sedans, 478 pick-ups, 129 SUVs, 181 hatchbacks, and 122 other vehicle images. Each image was collected using the 224×224 pixel resolution.
Link to download VTID1 dataset : https://data.mendeley.com/datasets/r7bthvstxw/1
Cite this dataset: N. Boonsirisumpun and O. Surinta (2022). Fast and Accurate Deep Learning Architecture on Vehicle Type Recognition, Current Applied Science and Technology, 22(1) (January-February 2022), 1-16. https://li01.tci-thaijo.org/index.php/cast/article/view/250863
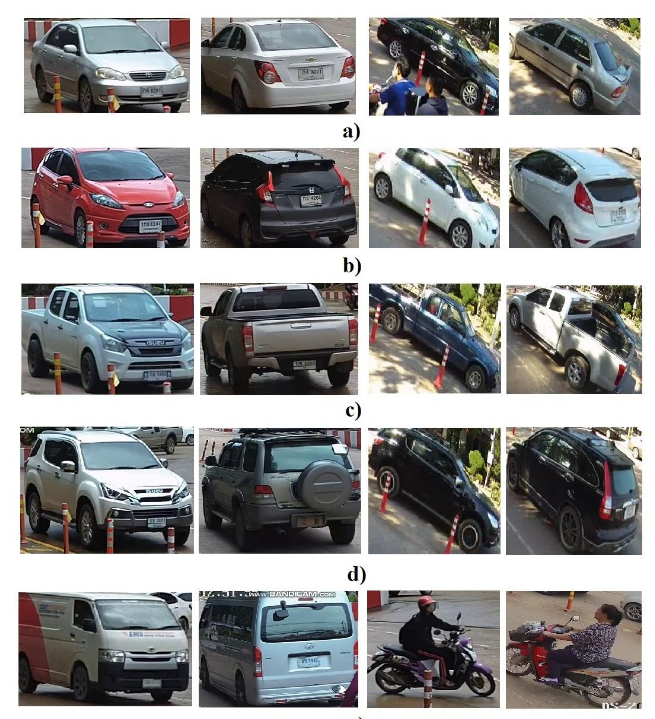
VTID2 Dataset
Vehicle Type Image Dataset (Version 2)
Narong Boonsirisumpun and Olarik Surinta
After creating VTID, the researchers decided to extend the collection process to create another larger dataset to add further diversity to the dataset in order to avoid data overfitting. Finally, the new dataset, called “Vehicle Type Image Dataset 2 (VTID2)”, consisted of 4,356 image samples that could be separated into five vehicle type classes as follows: 1,230 sedans, 1,240 pick-ups, 680 SUVs, 606 hatchbacks, and 600 other vehicle images.
Link to download VTID2 dataset : https://data.mendeley.com/datasets/htsngg9tpc/1
Cite this dataset: N. Boonsirisumpun and O. Surinta (2022). Fast and Accurate Deep Learning Architecture on Vehicle Type Recognition, Current Applied Science and Technology, 22(1) (January-February 2022), 1-16. https://li01.tci-thaijo.org/index.php/cast/article/view/250863
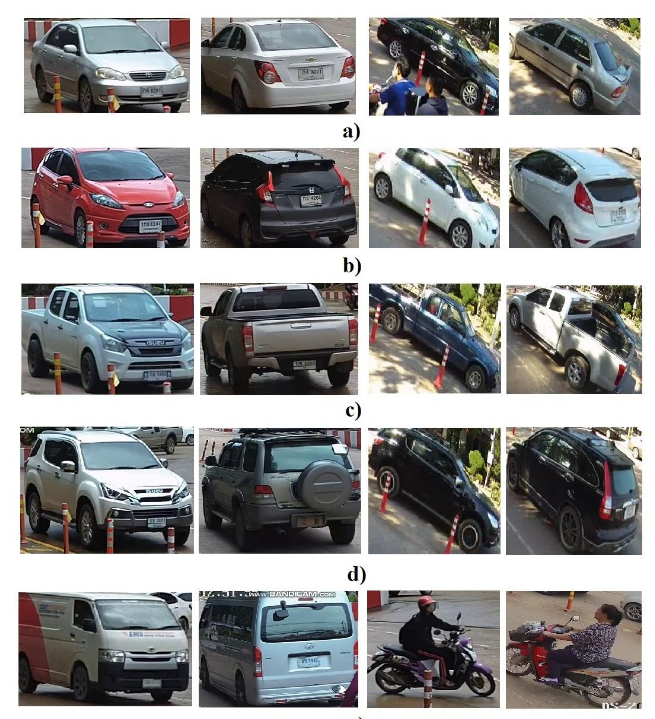
Multi-language Video Subtitle Dataset
Thanadol Singkhornart and Olarik Surinta
The video subtitle images were collected from 24 videos shared on Facebook and Youtube. The subtitle text included Thai and English languages, including Thai characters, Roman characters, Thai numerals, Arabic numerals, and special characters with 157 characters in total.
In the data-preprocessing step, we converted all 24 videos to images and obtained 2,700 images with subtitle text. The size of the subtitle text image was 1280×720 pixels and it was stored in JPG format. Further, we generated the ground truth from 4,224 subtitle images using the labelImg program. Also, the labels were then assigned to each subtitle image. Note that the number before the label is the order of the subtitle text image.
Link to download Multi-language Video Subtitle dataset : https://data.mendeley.com/datasets/gj8d88h2g3/2
Cite this dataset: T. Singkhornart and O. Surinta (2022). Multi-Language Video Subtitle Recognition with Convolutional Neural Network and Long Short-Term Memory Networks, ICIC Express Letters, 16(6)..
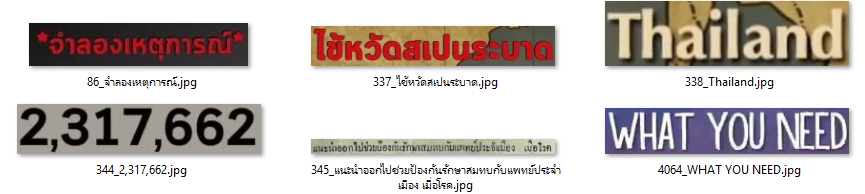
Vehicle Make Image Dataset (VMID)
Vehicle Make Image Dataset (VMID)
Narong Boonsirisumpun and Olarik Surinta
The vehicle make is the brand of the vehicle and mostly the name of the company manufacturing the vehicle. People easily recognize the vehicle by seeing the logo because of its unique design and is familiar to most people. This can help a machine do the same thing. By locating and recognizing the vehicle logo, it is possible for a computer system to classify the vehicle make by analyzing the differences in each logo and figuring out how to categorize them.
VMID was the collection of eleven vehicle logos in Thailand (Benz, Chevrolet, Ford, Honda, Isuzu, Mazda, MG, Mitsubishi, Nissan, Suzuki, and Toyota). The total number of images was 2,072.
Link to download VMID dataset : https://data.mendeley.com/datasets/8ssr6kptbx
Cite this dataset: Boonsirisumpun, N. and Surinta, O. (2022). Ensemble Multiple CNNs methods with partial Training Set for Vehicle Image Classification. Science, Engineering and Health Studies, 16, 220200011. doi: https://doi.org/10.14456/sehs.2022.12
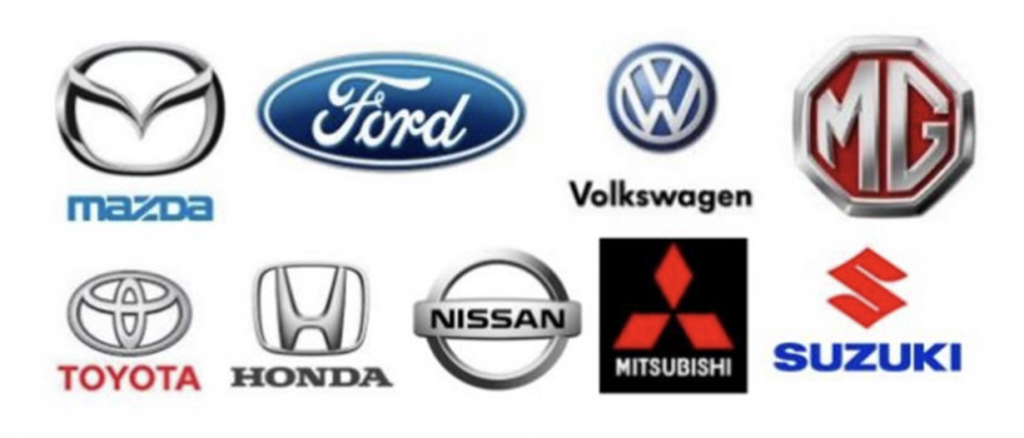
Example of the vehicle logo in Thailand
Count from October 23, 2021